The magic and traps of the predictive algorithm
Health, money, jobs, security. We are training machines to make personalized “modern horoscopes.” The amount of data we have spontaneously donated to the network seems to free us from so many risks. It does, but it has also created new ones that are difficult to control
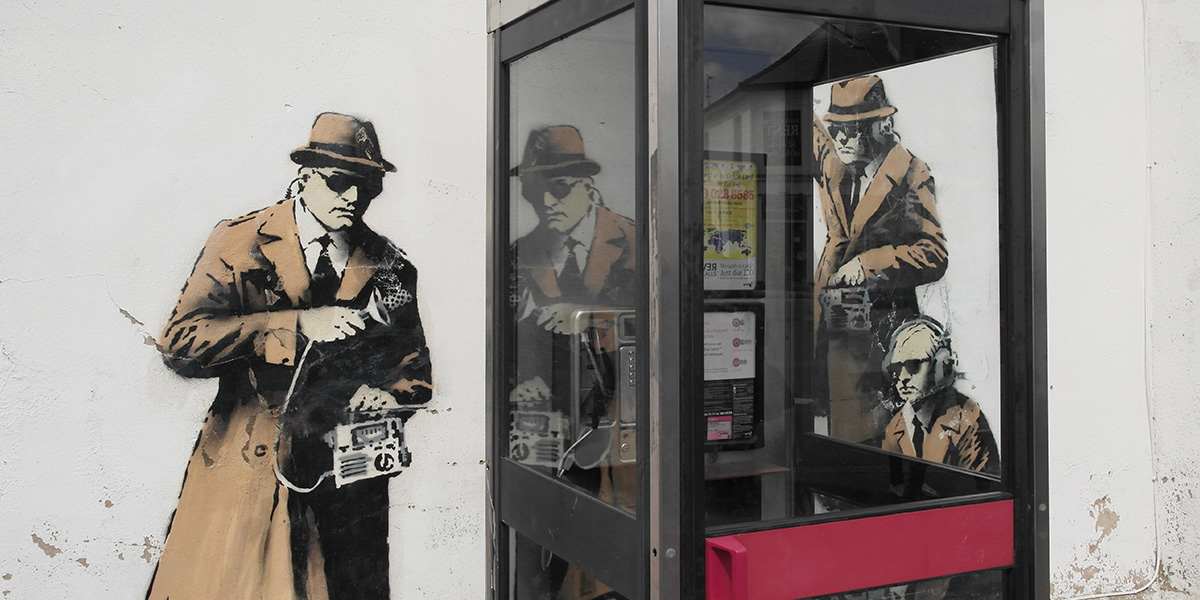
While technology, at its most sophisticated levels, is indistinguishable from magic, as Clarke’s third law reminds us, it is equally true that the 1980s saw the development of cyberpunk literature, which mainly highlighted the terrors, the pernicious aspects of technology, such as excessive surveillance. The visionary attitude of artists has been assisting us since the days of Charlie Chaplin and has often brought representations of future realities that we have easily confined to science fiction. Too easily, as in the case of Minority Report, the film that most of all dealt with the theme of the predictive algorithm, sticking some of the latest discoveries by scientists at MIT in Boston on the scene. There was, in the early years of the millennium, as there is today, something very difficult for the general public to understand, and at that time the film represented prediction as a supernatural talent of the precogs, who perceived in advance the bloodiest crimes.
While this was happening on the sets and in the publishing houses, however, the world was beginning to fill the Web with its own images taken with smartphones, and today there are 40 billion uploads. Suddenly everything started to become smart thanks to our data released into the web without any hesitation.
Twenty-two years after Minority Report and thirty years after the founding of Amazon, whose predictive algorithm (of our tastes and pockets) revolutionized global e-commerce, we now find ourselves with an exorbitant amount of data and with artificial intelligence that is tidying it up in so many infinite boxes, a great demiurge is producing alternative realities, which alongside enormous benefits brings new risks from which we need to protect ourselves.
Predictive police is, as in Minority Report, the dream of every "ordered" government. But the violation of sensitive data undermines many rights
If we talk about violating privacy for commercial and advertising purposes, the riskiest part of predictive algorithm hides in areas such as justice, financial management, and health-care. So, first we need to know the kinds of algorithms and machine learning that are being implemented, in environments and with rituals that sometimes have disturbing similarities to esotericism. Research defines predictive optimization as a decision-making process that uses machine learning, predicts future outcomes and makes decisions about individuals based on those predictions. Elements that hide traps at the time of their application. Here are some of them, codified by the European Digital Agenda and related to security and the administrative and judicial sectors:
- Predictive policing: enables identification of geographic areas where police should be deployed to guard public order;
- Welfare allocation: is already able to decide whether an applicant is eligible to benefit from the provision of a public service;
- Automated essay grading: uses data collected from the past to enable assessments in the present time;
- Traffic prediction: calculates traffic levels to estimate arrival time;
- Pre-trial risk prediction: collects past information on individuals to predict future detentions or court litigation.
Beyond the technicalities, the summary is that there is a degree to which automated decision making is dangerous. It also exists in our brains, clearly, and it is called cognitive bias or, in really poorer words, bias. Let’s consider a simple standard case that is often taught in investigative journalism courses:
- Mr. P.M. is found dead in his apartment on the second floor of So-and-so Street. At the time of the discovery, it was not possible to establish the cause, whether it was murder, accidental or natural death;
- Investigators do not reveal any clues but neighbors say that a strong smell of marijuana was often smelled from P.M.’s house.
- The reporter then learns that on the third floor lives A.V. a multiple convicted drug dealer and extortionist.
Having to write the article in a hurry, the reporter will make some connections between certain elements so as to write the story as it has been deduced: the neighborhood is run-down, the victim was unemployed and living hand-to-mouth, the person living upstairs can only be the first suspect, and the motivation can only be drug-related.
The correct definition of bias is: “A construct arising from misperceptions, from which judgments, preconceptions, and ideologies are deduced. Biases are often used to make quick decisions and are not subject to criticism or judgment.”
Well the same happens, with the computational capacity of today’s machines, for algorithms as well. What was thus born to make simple statistical comparative investigations on the collection of data recorded in the past, has made a shift in recent years that allows it to provide predictions on hypothetical future trends.
The level of impact this has on people’s lives obviously changes depending on the sensitivity of the scope, because it is clear that if this profiling is produced to sell more diapers, that is one thing; if, on the other hand, it is to be used to arrest someone, everything changes.
Predictive policing
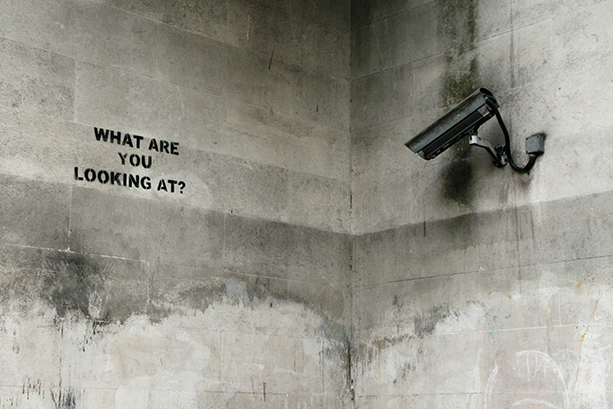
Some of the artist Banksy’s work on the theme of surveillance.
In the 1990s, the New York Police Department initiated a policy that has today led the Big Apple to be one of the safest cities in America. In 2018, there were 289 homicides in the city’s five districts. The homicide rate, 3.31 per 100,000 people, is the lowest ever recorded in the previous 50 years.
It was much different in 1990, when the murder count was 2,245, about 31 per 100,000 people (the population by the way increased significantly in the following 28 years).
To portray this sense of insecurity, the New York Times wrote, “New York already looks like a New Calcutta full of beggars. Crime and fear make it look like a New Beirut. Safe streets are essential, getting out and walking on them is the simplest expression of the social contract. A city that fails to hold up its part of that contract will suffocate.”
In 1993, Rudy Giuliani nominated Bill Bratton, a former Boston cop, as head of the NYPD. He realized that his new department did not focus at all on crime prevention. Officers thought that in order to do their job, crimes had to have already happened.
The police did not have access to data, so the department began to develop statistics. Consultant Jack Maple invented timely intelligence, which was the concept that real-time, up-to-date data were needed to prevent crime. This was not at all obvious at the time.
Whether this was decisive is not proven, but with the data-driven approach, crime dropped. Today, if we talk about security, predictive algorithms are an outgrowth of that approach taken by the NYPD and other agencies around the world.
However, it is also known that the assumption that a thief will decide not to steal wallets on Thirtyfourth Street because he knows that the police use the predictive algorithm is not credible.
Phillip Atiba Goff of New York University’s Center for Policing Equity, when asked continually by newspapers and technology sites about the real effectiveness of a predictive approach to police action, replied pointedly, “Algorithms only do what we tell them to do.” And, so, what do we tell them to do in the age when law enforcement has 40 billion photos (probably obtained in an often questionable way) to build identikit and cameras set for the most accurate facial recognition? The temptation to create true predictive policing has been, and to some extent is still, very strong. As New York Times journalist Kashmir Hill witnesses in her book Your Face Belongs to Us (in Italy published by Orville). Yes, too many botched and missteps have led to the arrest of innocent people. The list of episodes told by Hill is disturbing, and the journalist has abandoned smartphones and now uses an old Nokia model. Which says a lot about the risks we will have to identify.
The predictive algorithm in economy
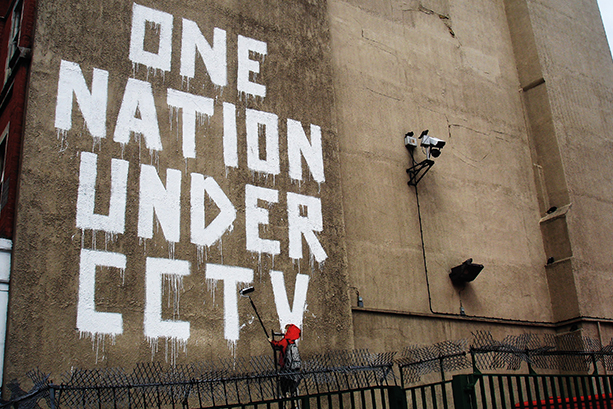
Where it is perceived as an aid to make sales of goods and services increase, predictive algorithm excites the spirits of entrepreneurs who do not see or pretend not to see the risk of customer manipulation. Because predictive AI presents itself as a golden goose: what could be better than guessing and predicting customer tastes?
The technology is there, machine learning is increasingly accurate, but what companies often lack is foresight in identifying areas that can be improved by Predictive Analysis.
Predictive Analysis is certainly useful in CRM (customer relationship management), in activities such as marketing campaigns, sales, customer satisfaction, aftermarket. The purpose is to analyze customer behavior to determine how they may react to different prompts or to see if there are recurring patterns of behavior that can be exploited for business purposes. It is in this field that many machine learning techniques such as classification or clustering algorithms find application, to segment customers, perform churn-analyses (which measure the rate at which the public gives up on a product or service), assess retention (the percentage change in loyal customers) or simply to increase effectiveness in sales and marketing through optimizations and tailor-made measures. All of this is of little concern because it does not seem to touch the core of informed customer choice. Yet several ethical issues may arise, for example in assessing the impact on production chains, their sustainability, choices to outsource production, in countries where quality controls are less tight.
Another area in which predictive algorithms are successful is in decision support in incomplete information contexts, in which some degree of uncertainty generated by human choice remains. Fed with meaningful data taken from the past, algorithms are indeed able to determine what kinds of actions have been successful in the past and apply the right decisions to similar cases that arise in the future. Some of these choices could even be, at least in part, automated. As in the case of deciding whether or not to discard a product because it is defective. It is clear that there will be errors in the process of refining this technology toward perfection. Will we be able to predict which ones and how to deal with them?
Finally, for companies that offer a wide range of products, Predictive Analysis can help offer deals with the goal of selling several different products at the same time, an action called cross-selling, and the best example of this is the offer of a smartphone combined with a digital watch. With an up-selling strategy, on the other hand, consumers can be led to choose the products of higher value and higher price. However, the consumer will need to be aware enough to really evaluate value for money, and this, in e-commerce disintermediated by people and places of purchase, is not easy to achieve.
Predictive Analysis on consumer behavior is thus useful in coming up with ideas for product combinations, communication strategies, and seasonality of marketing. And here, however, there is an unresolved paradox, although the increasing computational power of the machine may solve it: consumption trends are standardizable according to population, age income, but they are also customizable by acquiring more and more sensitive data, which themselves have a market, and the recent scandals that broke out in Italy in late October 2024 should push us to better investigate the rules of the game.
Finally, there is the area of preventing fraudulent behavior and risk management: the financial services and insurance sector is certainly among the most affected, and many companies already adopt Predictive Analysis solutions that identify fraudulent transactions and fake information. When it comes to risk management, however the goal is to reduce or eliminate sensitivity to events that could harm companies. Predictive Analysis, in this case, provides the probabilities that are linked with each risk factor so that the most appropriate measures can be taken.
The most sensitive of predictions: health risk
Among the uses of predictive algorithms, those designed for the medical field definitely point to the goal of improving the accuracy of diagnoses and the effectiveness of treament for particular diseases. And here, as indeed in all scientific applications that analyze data, the key concept is that of data hygiene. In health-related decisions, the right selection and aggregation of data is the first step toward achieving the result. Hygiene of the data is also the search for objectivity without exposure to the ideological bias of those who may lead the interpretation. Let us take a case study that is quite frequent but never really addressed. There are private clinics that claim very low mortality rates of their patients when compared with the public health service. What is not mentioned is that these clinics do not have terminal wards, as they do in the public. And so people can be led to believe that the private is of higher quality.
However, predictive medicine has made progress by optimizing genetic information, bio-markers and personal data. Today predicting the risk of developing diseases such as heart disease, cancer, diabetes and more is easier. Preventive measures or more frequent check-ups can then be taken to reduce the risk or to promptly diagnose the disease. Data that will provide patients with specific advice and guidance instead of a generic prevention roadmap. This means that a treatment may be considered appropriate if it leads to positive results for that specific patient, even though it may not be effective for an only apparently similar group of patients. It is clear, however, that this path requires more economic resources. Could it be for everyone? AI can analyze huge amounts of clinical and diagnostic data to identify patterns, trends, and correlations. The way humans will handle these powerful innovations will then impact on the possibility of creating efficient treatments. That is why protection from risk needs to come through good regulation as soon as possible.
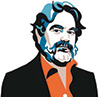
Davide Burchiellaro
Deputy Head of Content at Linkiesta, has worked for many years at Panorama,
headed Marie Claire digital and is now studying different and philosophical viewpoints on AI.